While events are a mandatory part of SIEM, as the acronym implies, correlations are not. That said, they became a synonym to the term SIEM. Should they be considered a core element of SIEM? Are they useful at all? To answer that we first need to examine what they are and what they can be used for.
Correlations vs. Query and search
Correlations are used by the SIEM as a key analysis method for the collected events, but it is not the only method. The archrival methods are query and search, both focusing on analyzing a batch of events at a later time rather than analyzing events as the come. The pros and cons of each method are key to understanding SIEM products and current dynamics in the SIEM landscape, most notably the move from SIEM to “big data”.
In this article we will focus on what correlations can do, what they are useful for, and what they are not ideal for. A later article will address search and query and will enable us to compare both.
Event egress processing (a.k.a. “correlations”) functionality
There is no standard for correlation logic language – often called “rules” – and each SIEM uses a different paradigm and terminology for creating those rules. Moreover, correlation capabilities are often distributed within a single SIEM solution between different modules: some at the collectors, some at intermediate aggregators and some at dedicated central correlation engines. The following sections try to avoid the actual implementation details to describe common functionality that correlation engines offer for processing and analyzing events.
Filtering
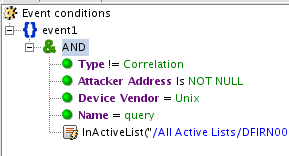
An ArcSight filter
Starting with the simplest implies we are the furthest one can get from the dictionary definition of correlations, however just dropping uninteresting events is an important event processing stage that streamlines the downstream processing of events and ensures less information overload.
Filtering may be based on data in the event, for example:
- A successful connection through a firewall is deemed unimportant.
- Specific event types sent by the source might be useless for the SOC.
Filtering can also be based on more complex conditions that might require the filtering to be performed after other correlation functions. An event might be discarded only after it is enriched with additional data which ensures it is OK. For example, a policy change in a firewall might be discarded if done by a valid firewall administrator or done on a test system, determined by looking up the role of the user and the firewall respectively. Even a “join” condition between events might be used to discard the events: in the firewall example above a preceding event might ensure that this is a valid change window.
In practice most simpler filtering is done early on in the event life cycle at the collectors and at times even at the source device: for example, Windows can preselect which events it sends to an event collector. This ensures filtering is distributed, saves network bandwidth and saves processing load from central SIEM servers.
More complex filtering needs to be done on the SIEM server. While many SIEMs do not have a concept of dropping events (and probably should have), they do filtering using “filtering in” rather than “filtering out”: the correlation logic selects the events that are useful and emphasizes them, usually by creating a correlation event that is included in the main event monitoring channel.
Enriching
Events as sent by the source are usually minimalistic in nature and to be useful for further analysis, automated or manual, additional information should be added to them. Common areas for enrichment include:
- Host name resolution
- Geographical information for IP addresses
- Account name (system specific) to identity (organization wide) resolution
- Adding user information such as role and department
- Adding asset information for the devices involved in the event such as role (server, type of server, desktop etc.), business criticality and owner details.
- Looking up the reputation of IP addresses and web sites reported in the event.
- Assigning a priority taking into account the event and enrichment information.
Much of the enrichment information can be categorized as “context” which the SIEM has to import from dedicated sources or learn from the event stream. A special king of enrichment is based on joining multiple raw log entries, each containing partial information, into a single richer event.
Those simpler enrichment capabilities can be implemented at the collector layer. More advanced enrichments rely on data that is derived from previously collected events. An example is IP to user attribution: assigning a user name to events that include only an IP address. This is done by keeping a session list connecting users to IP addresses based on periodical events that include both user and IP address information such as login events.
Those simpler enrichment capabilities can be implemented at the collector layer. More advanced enrichments rely on data that is derived from previously collected events. An example is IP to user attribution: assigning a user name to events that include only an IP address. This is done by keeping a session list connecting users to IP addresses based on periodical events that include both user and IP address information such as login events.
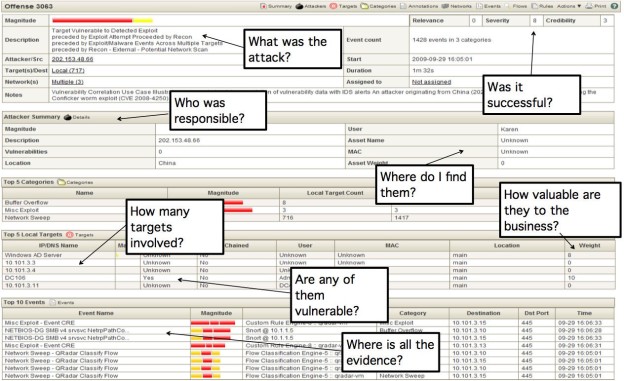
A QRadar offense exemplifying enrichment data such as Geo location and business priority
Aggregating
The simplest feature which focuses on “correlating” multiple events is aggregation: connecting together a number of similar events. Aggregation has two main use cases:
- Reduce the event load by reporting once large amounts of repetitive information, usually by adding to the base data a count as well as time stamp for the first and last occurrence. This is commonly done at the collector layer.
- Identify incidents that are manifested in the repetitive nature of the events such as port scans or brute force attacks.
An important consideration is that while event reduction usually aggregates very similar events, incident identification might require more complex conditions. For example, a port scan is usually identified by access to different ports rather than to the same port.
Joining and sequencing
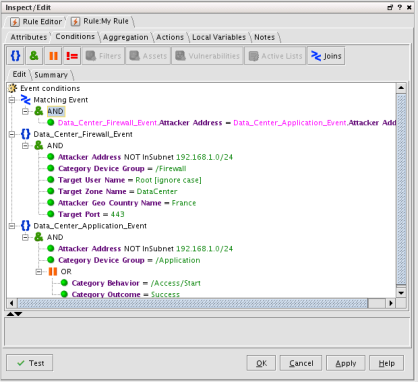
An ArcSight join rule
Lastly, the holly grail of correlations is actually grouping a number of events that presumably tell a story when linked. The brute force mentioned above for example might be more interesting if the repeated login failures were followed by a successful one. Sequencing is a variant of joining that required an order between the grouped events.
While joins are mostly associated with incident detection, they are useful also for filtering in or out events as demonstrated above. In many cases they can also enhance, or correct, events by grouping a number of related raw events (or log entries as discussed in “Understanding SIEM: Events, Alerts and Logs”) to one more useful event that includes all relevant data. This latter user case is the only variant of “join” that is commonly done at the collector level.
The plumbing
Since correlation rules implement logic, they require a “programming” toolset. Leaving aside the traditional programming tools such as variables and functions, the following are important aspects of rule programming that are characteristic to correlation rules.
Actions
The discussion above focuses on conditions. However just matching incoming events would not be useful. Upon matching, the rule has to be define actions to perform. Those actions usually fall into the following categories:
- Raise an alert or notification to the SOC operator. This may take the form of an external alert using an e-mail or a pop up, or an internally by creating an incident (case or correlation event in ArcSight, offense in QRadar) and listing it in the SOC incidents channel.
- Update a dashboard, a live alert channel or some other graphical representation. For example, a rule might switch a semaphore on or off on or update a counter on a dashboard to inform the operator on overall security posture.
- Update the event, usually with enrichment data.
- Generate yet another event, often called “correlation event”. While this can just be used as an internal alerting, it is commonly used to create complex correlation logic as this new event would itself be processed by the correlation engine.
- Updating context information used for enrichment, usually in the form of updating lookup tables. This is often used to manage state lookup tables based on incoming events, for example to associate an IP address with a user based on logon events as discussed above.
- Execute an external action, for example using an external command or a REST API call. While an external action can implement any additional logic, the most common uses are for automated remediation and for integration with external systems such as ticketing systems.
Triggering
While for simpler rules triggering actions is simply based on a successful match, more advanced correlations such as joins and aggregates complicate things. Specifically, how would the rule behave after an initial match is found? For example, if a rule tests for 50 events of some type in a 60 seconds window, how would the rule behave if 51 events are received within the window frame, or a 101? Or a 100 in 90 seconds?
To address that, rules may offer multiple triggering options: on first or subsequent events matching the condition, on first or subsequent threshold matches, periodically while the evaluation window is still open and when the evaluation window expires. A use case for such elaborate triggering might be to turn on and off a dashboard semaphore on a first threshold trigger and window expiration trigger signaling that the complex condition is active or not respectively.
What correlation engines do not do well?
The rather technical discussion above can hide the downsides of correlations as an analysis method for events, however correlation rules are not best suited for some use cases:
Signature and IoC matching
Users often try to use correlation rules to find indicator of compromise (IoCs for short) in the event stream. Examples might be ill-reputed URLs or SQL injection signatures. This requires matching one or more event fields to a long list of strings or regular expressions, which correlations engines just do not do well.
Correlation rules can usually compare a field as a whole to a list of values using a lookup. But while feasible, no correlation engine to date performs well search for a partial match of multiple signatures or regular expressions within a field. This is the realm of intrusion detection systems. Moreover, such a comparison is prone to evasions, something that a dedicated intrusion prevention system will mitigate.
It is worth noting that query and search are not much better at that.
Baselining and analytics
One of the biggest limitations of correlations as a modern-day analysis method id their limited usefulness for analytics. Most analytical models take into consideration a large amount of data which does not fit well the one event at a time nature of correlation rules.
A good example would be baselining: while a correlation rule can update a state table used for baselining, this is cumbersome to implement. It is often much easier to run a periodical query against collected events to create a baseline.
It must be noted that correlations rules can be used effectively to test an event against a baseline and offer the benefit of providing real-time results. QRadar for example uses a dual approach for its behavioral correlation rules: search is used for building the baseline while a real time rule is used to evaluate events against the baseline.
Performance considerations
Correlations are a rather optimal method for analyzing events as they consider each event individually, in memory, as it is received by the SIEM. In many cases utilizing correlations well is far superior performance wise to search based analysis and may also provide more correct results. For example, attributing event to a user using an IP to user lookup table is more accurate and efficient if done for each event individually at the time of receipt.
That said, correlations rules have performance implications to consider. The core challenge is the impact of the automated context management required for join and aggregate rules. Long time windows for such rules may require a very large number of open contexts (or “partial matches”). For example, a rule that would try to determine a “slow” port scan over a time window of a day would have to keep an open context for each source IP from which communication was received by the firewall for a day, something that can easily hog the resources of a SIEM server. The risk is that this performance hit is hidden from the cursory user. A more resource friendly solution would be to maintain explicit context by using granular rules that explicitly update a state table. The price is the development complexity discussed next.
Simplicity
Correlation rules use the event driven programming paradigm. When an event is received it is evaluated and triggers actions. Users often find it hard to grasp this paradigm opting for the more traditional procedural programming available using search and query analysis.
As an example, when faced with the requirement to match an events with a list of ill-reputed IP addresses, users will often use a report that based on a join query (or a lookup search) rather than build a rule to perform the lookup when the event is received. The latter will provide real time results and utilize less computing resources but is less intuitive to most.
Built in conditions that evaluate multiple events such as joins and aggregates hide the complexity from the user but often introduce hidden side effects such as the performance issues or the triggering complexity discussed above.
What are they useful for?
The discussion so far focused on the technical aspects of correlation rules. The goal was to clear some of the mystification built by the traditional use case driven description of correlations which is often marketing rather than technically driven.
Now that we understand the functionality provided by correlation rules, it is the time to re-visit the use case aspect and discuss how correlation rules actually contribute to security.
The first use that comes to mind is threat detection. Whether and how “joins” and “aggregates” contribute to better threat detection is a broad discussion left to a subsequent article.
However, correlations rules are more immediately useful for other use cases critical to efficient SOC management with focus on threat prioritization, investigation and mitigation rather than detection. Among those are:
- Enhancing events, for example by joining a group of related but partial events into one event that has all needed attributes.
- Filtering (in or out) events to ensure the operator is not flooded.
- Prioritizing the remaining events using event data and context information to optimize event handling.
- Enriching events, both to support filtering and prioritization as well as to provide more information for the analyst to assess and investigate an event.
In a following article we will discuss how search and query work and see which of those use cases better suit correlations (i.e. traditional SIEM) and which suit search (i.e. Splunk).
Nice blog. Here I found valuable information on cyber security and importance of cybersecurity SOC. Thanks for sharing.
ReplyDeleteBy network security management means finding the attack in network security and implementing firewalls to protect it from several risks by implementing best cyber security solutions.
ReplyDeleteSIEM security develops a safe and secure environment for the information log in the system and ensures that it is managed and ensure that it is secure and safe from multiple attacks that occur within the system.
ReplyDelete